Avoiding False Triggers in Trail Cameras
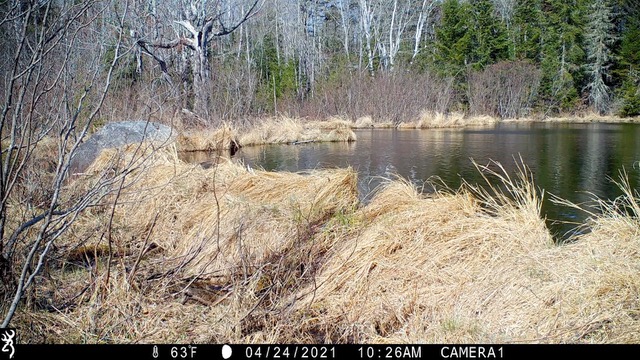
False triggers, resulting in photos and videos with no animals, are the bane of camera trappers everywhere. The PIR sensors in modern trail cameras do a pretty good job at triggering for animals. Unfortunately, they will also trigger for other things, like waving vegetation, a moving camera mount, and (in some circumstances) ripples on water. In this post I will cover the underlying causes of false triggers, as well as some practical tips for avoiding false triggers in camera trap sets. As in the cover photo, I will use real-life data, photos, and video taken from our too-large collection of captures without animals.
How PIR Sensors works
(Unlike the sensors in Star Trek) PIR sensors don’t (fundamentally) detect “lifeforms”. They detect changes in temperature which appear between certain regions in the sensor’s field of view. Mammals regulate their temperature, which is very likely to be different from the background temperature of other objects in a scene. Thus, an animal passing in front of the camera will cause a change in temperature in different regions of the scene as it moves. This change in temperature is detected by the PIR sensor. When the change exceeds some threshold, it triggers the camera.
False triggers occur when something other than an animal causes a change of temperature within the PIR sensor’s field of view.
For a more detailed look at PIR sensors, see References a the end of this post.
Using File Metadata to Gain False Trigger Insight
Sometimes it’s hard to tell, just looking at a sequence of “empty” photos or videos, what exactly caused the false trigger. I’ve found it useful in these cases to look at the file meta-data. Specifically, the date and times each photo/video was taken (assuming the date and time on the camera are working correctly).
I created the graph below by copying the results of a “dir” (list directory) command on SD cards retrieved from 8 cameras from a recent deployment in Maine. Then, I pasted these into Excel. The graph shows the cumulative number of triggers as a function of date. These 8 cameras had been in the field since early April 2021. We retrieved them in late September of the same year. Critically, this period includes the peak of spring growth. You an see this definitely figured into the success (or failure) of some of these sets.
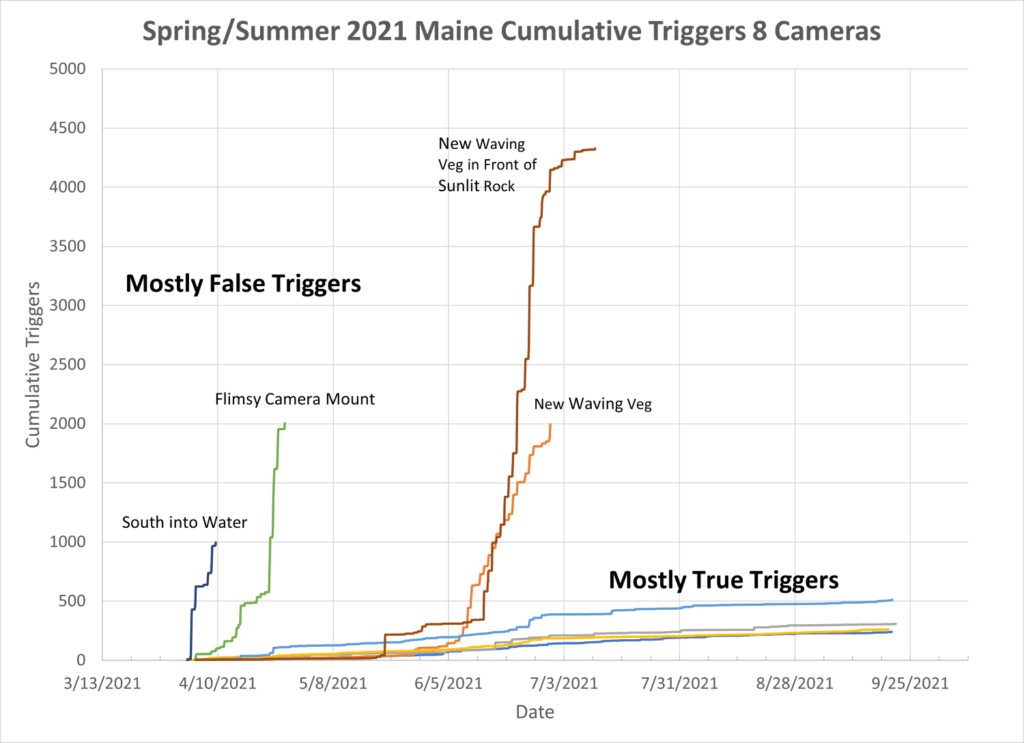
The graph clearly shows cameras which had an excess of false triggers. These are the 4 lines toward the left of the graph which shoot up, rapidly accumulating thousands of triggers. Careful examination of the slope of these lines shows that these cameras were triggering at near their maximum rate (yet not so much as to suggest, “run-on triggering”, described in How (some) Trail Cameras Fail). The other 4 lines have a nice, gentle slope upward. They have few vertical jumps, and contain a large fraction of “true” triggers of some type.
I have labeled the lines with high false trigger rates with their primary cause. I’ll discuss how to avoid these in the next section.
Avoiding False Triggers
Here area some tips for reducing false triggers. The video below gives examples of not following some of these rules from the same set of 4 “false trigger” cameras in graph above. Sorry — no animals.
Sturdy Base
The PIR sensor can easily trigger if the camera moving. If the camera moves relative to the background, perhaps because it is mounted to a swaying tree, the PIR sensor will detect “moving heat” and will trigger. Avoid this by securely anchoring the camera to a large, sturdy tree. If a small tree is the best available, and if the site allows for it, cutting off the top of the tree just above the camera can substantially reduce camera movement due to wind. In the case of “make do” sets (see The Art of the Treeless Set), make sure that the ad hoc camera support is sturdy – not just when you set it up, but over the change in seasons.
A second problem can arise if the camera is loose inside a security box. Even a little movement caused by the wind can induce false triggers. We ran into this problem when we started using security boxes, screwed into a tree, and a padlock only. Without a cable lock securing the camera, there is a couple of mm “play” in the camera. It’s easy enough to also use a cable lock. Compressing a thin piece of foam rubber into the space between the camera and the security box also works. In the example in the video above, we made a make-do set with a security box and just a padlock. You can see that as the wind blows, the camera pivots up and down just enough to set off the trigger.
Avoid Sun Facing Sets, Especially into Water
There several good reasons for not facing your camera southward, into the sun. Front lit photos and videos are often higher quality than those which look into the sun. Further, having the PIR sensor face the sun can also increase the rate of false triggers. We’ve found this to be especially true with cameras facing into the sun, and at a body of water. In the video above I have an example of such a set in which IR radiation from the sun was reflected into the PIR sensor by wind-blown ripples on a beaver pond. The ripples created apparent changes in heat in the scene. These triggered the camera nearly constantly during the day, almost from the day we set it out.
Note “not pointing the camera into the sun” generally means pointing it towards the north in the northern hemisphere, and towards the south in the southern hemisphere. To be sure, use a compass (including the compass app on a smartphone). Thanks to Chile-based BernardoS for pointing this out in comments on original post.
Beware the Sunlit Rock Background
A rock background can provide a dramatic backdrop for many animal photos and videos. It is especially useful for night-time color shots because it reflects some of the light from the flash to create a brighter photo or video. Unfortunately, a varied rock face facing the sun can result in a background of different temperatures. Even small waving vegetation between the camera and the rock can cause the PIR sensor to trigger. Our most successful rock-backed sets have been in well-shaded areas, with practically no vegetation. Unlike the set in the photos below.
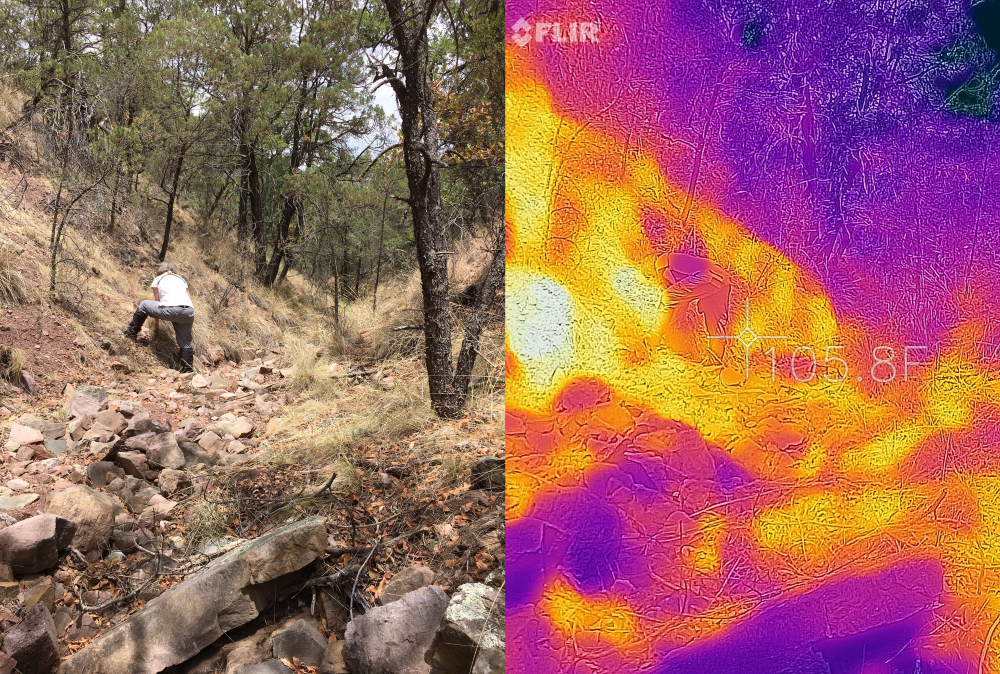
Avoid Growing Vegetation
Leafy green vegetation blowing in the wind can alternately shade and expose background objects with different temperatures. We carry a pair of clippers and a small saw to clear away vegetation from the set. This is tedious, but quite effective. Problems arise in sets pruned in advance of a major growth spurt in the surrounding vegetation. In this case, it’s best to look for spots free of signs of earlier growth (e.g. tall, dead grass). Another strategy is to visit and update sites after a major growth spurt. The timing for this varies by region, of course, but the basic rule is that vegetation growth happens in early spring.
Reduce Sensor Sensitivity
Newer cameras often have a setting for adjusting the sensitivity of the PIR sensor. That is, the threshold in temperature difference required to trigger the camera. For example, on Browning Recon Force Advantage and Spec Ops Advantage (BTC-7A and BTC-8A, resptively), this setting is listed under:
- Motion Detection: Options are for “60 ft” or “80 ft”
These options might as well be labeled, “low” and “high” sensitivity, respectively.
Unfortunately, although reducing sensitivity will decrease the number of false triggers described in this post, it is often not nearly enough. Moreover, reducing sensitivity also decreases the chances that the camera will trigger on animals.
Limit Operating Hours
In many case, the time at which animals are active is not the same time of day with the most potential for false triggers. Wind often reaches a maximum more or less in mid-day. By this time, the sun has heated up some objects, and not others, creating perfect conditions for false triggers. If your target species are not active during this time period, one solution is to program the camera to “turn off” for certain times during the day. Not all camera models support this feature.
Below is a chart that shows the relatively frequency of true and false triggers by time of day for a set in Maine accrued between April and September. Setting operating hours for the camera to by outside of 9:00 am to 5:00 pm would have substantially eliminated false triggers on this set. Unfortunately, you might also miss exceptional appearances of your target species during this time. Since these shots — like a lynx in the daytime — are highly prized, disabling the camera only during false trigger peak time is a better strategy. In the example below, we would have seriously reduced the number of false triggers by disabling the camera between 11:00 am and 1:00 pm.

The exact distribution of false trigger times during the day will vary with camera location, how the camera is facing relative to the sun, and other factors.
As far as when animals are active, the development of so-called “animal activity curves”, increasingly based on camera trapping data, is subject of current research. See References below. Knowing exactly when your target species, in your location, may require some of your own research.
Recovery Delay
Most trail cameras have a setting which allows you to adjust the minimum time between successive triggers. One can reduce the number of false triggers a camera records by increasing this time. Thus, in the worst case, a camera that triggers at most every 5 minutes during a windy day will take far fewer videos than one that can trigger every 20 seconds. Unfortunately, increasing the recovery delay has a downside for true triggers. With a delay of 5 minutes, for example, a camera set to take a 20 second video will only capture the first 20 seconds of an exceptional 2 minute animal encounter. One could compensate for this by increasing the length of videos. Unfortunately, this also causes false triggers to consume more battery and SD card resources.
Maximally Provision and Check your Camera
When it’s not possible to follow the rules above, one can maximize the chances that the camera also catches at least some true triggers by:
- Using high capacity batteries at full charge: e.g. LiFeS2
- Using an SD card large enough to exhaust the batteries: 256 GByte for Browning Recon Force Advantage (BTC-7A) when using LiFeS2 batteries and taking “ULTRA” resolution video.
- Visiting frequently to “freshen” the set (especially during times of growing vegetation)
Conclusion
Sifting though false triggers is time consuming and frustrating. Missing an opportunity to capture an animal because false triggers have filled the SD card is even more frustrating. In this post, I’ve attempted to summarize techniques to avoid false triggers in trail cameras. If you have used these techniques successfully (or unsuccessfully!), or have others, please comment below.
References
Peter Apps, John Weldon McNutt, “How camera traps work and how to work them,” African Journal of Ecology, 2018.
Rowcliffe, J. M., Kays, R., Kranstauber, B., Carbone, C., & Jansen, P. A. (2014). Quantifying levels of animal activity using camera trap data. Methods in Ecology and Evolution, 5(11), 1170-1179.
Lashley, Marcus A., Michael V. Cove, M. Colter Chitwood, Gabriel Penido, Beth Gardner, Chris S. DePerno, and Chris E. Moorman. “Estimating wildlife activity curves: comparison of methods and sample size.” Scientific reports 8, no. 1 (2018): 1-11.
Great article. Personally when I install a new trail camera in a place with potential high false triggers, I try to set it in a conservative way, this is with long intervals (like 25 sec), photo mode, low megapixels. Then I check it a couple of weeks later, if it’s all good then I usually change it to video or a better quality photo with low interval (like 5s). After that, I can let it for several months with confidence. I mostly use trail cameras for research so the camera has to work at all times, loosing trail camera time due to battery or sd card storage has to be avoided at all cost. BTW, here in the southern hemisphere we have to place the camera facing south. Cheers from Chile.
“Trying out” a set certainly makes a lot of sense. And thanks for confirming the location of the sun for our southern hemisphere readers 🙂 (I suspected this was true, but couldn’t convince myself without a picture). I will add to the post. Thanks for reading and commenting!
Great stuff. Thank you. We are out in the Western US and the wind is a steady companion on the open prairie. There are indeed such places where there is nothing one can do to avoid those false triggers and yes, they are usually in the middle of the day. Almost like clockwork.
Glad you found it useful. For “clockwork” triggers, have you tried reducing operating hours? Or, like us, do you always find yourself hoping to find something really good in the haystack of false triggers?
Thanks for this article. I thought PIR sensors primarily picked up movement, not realizing the importance of temperature change and its effect on the PIR sensor. With respect to your suggestion to cut off the tree just above where the camera is attached makes complete sense; however be mindful that the camera and lock may be stolen simply by pulling them off the tree! I had locked one of my cameras on a tree with about a 2.5 inch diameter. Someone bent the tree to break it, and slipped my camera and lock off.
Something I’m very curious about is why moving water doesn’t trigger a camera, even when the camera is set close to the water, yet when the camera is set close to moving vegetation it’s triggered incessantly. Is this because there aren’t temperature changes with moving water, but there are with moving vegetation? And, if this is the case, I wonder why there aren’t temperature changes with moving water….
Sorry to hear about you camera 🙁 You’re absolutely right about the danger of mounting cameras to small trees. Especially if you cut them to keep them from blowing around 🙂 These days, we deploy our cameras only in steel lockboxes. We have also taken to screwing these into the trees through the mounting holes in the back. A cable lock through the side holes and/or a padlock through in front secures the camera (and prevents access to the screws). This makes it much more difficult for a thief to remove the lock box or camera. It also makes it easier to check the camera without requiring us to re-aim the set. Since the lock box is screwed in and doesn’t move, we just pop the camera out and then back in. That being said, nothing works 100% against theft.
Water is tricky because it has lots of interesting behaviors. It flows, obviously, and yet relatively high thermal conductivity means all of the water in a stream passing by your camera is likely at the same temperature, so there is no “moving changes in temperature” to trigger the camera. On the other hand, it can also lap up against non-water edges, possibly at different temperatures, leading to changes in temperatures as water covers, then recedes, which could cause triggers. Finally, water is a good reflector of the Near IR radiation detected by the PIR sensor. The surface of flowing, or blowing water, can therefore act as a rippling mirror, causing a static thermal background to appear to the PIR sensor as “moving” temperature — another source of false triggers. Having said all this, your question begs some “hard data”. I’m going to take my thermal imaging camera out to our next water set, and collect some data. Perhaps this will make it into a future post just on water!
Thanks for reading and commenting.
Thank you very much! I would be very interested in seeing a post just on water. Clearly, it’s a very interesting element when it comes to trail cameras. I currently have a camera set up by a flowing river, just off shore (hoping to get grizzlies feeding on salmon). Last year, when I set up a camera along the river, I was surprised and pleased to not have gotten any false triggers. The current (no pun intended) setup has been there for about a month now and I’m hoping to get back to it within the next few weeks, however, I’m dependent on someone taking me in their boat. Nevertheless, when I get the camera, I’ll let you know if I get any false triggers and also if I don’t! Thanks again, Mary Beth
Sounds like it will be an exciting retrieval — good luck!
Hi all
I think with excessive false triggering there’s another problem/risk that arises: In a situation when the cam captures videos nearby constantly (seamlessly) because of false tiggering, any animal activity during this period of time will be recorded on a video that’s already running. There’s no triggering of the cam – the cam is already running. This means that the animal activity is most often not visible within the first few seconds of the video containig it. As inspecting the whole duration of every single video is extremely time consuming, one tends to check only the first few seconds of each video for animal activity, and to delete any video that shows no action within this short period of time. So even recorded animal activities will get lost. Hmmm… I wonder wheter anybody knows a program/software beeing able to run a batch check running through a large heap (hundreds) of videos for any unusual (animal) movements, and beeing able to create a list of videos containing such an “anomaly”. Having something beeing able to do this would massively reduce the risk to loose precious captured animal movements, and it would save all of us a lot of time we can spend for better things by scanning through mountains of useless videos.
What do you think? Please let my know, I’m curious 🙂
You are right! We have often had the experience of thinking there might be camera trapping gold hiding among gigabytes of false triggers. More generally, it would be a great if there were a way to automatically find all videos with animals, perhaps sorted by species and “quality”. I believe the technology exists for such a system. For example:
* MegaDetector: Is a highly accurate, open source, AI model for finding animals in trail cameras (much more subtle than finding animals in photos taken by people). https://github.com/microsoft/CameraTraps/blob/main/megadetector.md While MegaDetector doesn’t give a “quality” metric, it does provide a bounding box for each animal detected, and a probability that it’s identification is right, both of which could be used to inform a crude “quality” metric.
* MegaDetector runs a frame at a time. This is fine for still photos. For videos, one would have to split the MP4’s into a sequence of frames, and process each frame through MegaDetector. I am aware of some experimental models that take information from multiple frames (the way we do when we flash back and forth between frames) to improve predictions, but not one that operates on seconds worth of video.
* MegaDetector is designed for accuracy, and it’s very good, but it requires a fairly beefy server running with a GPU or two. I’ve had luck running it on the free version of Google Colab, though it runs even better on the (USD $10/month) “professional” version.
* The trick is then to get the data from the SD card out to the cloud where Megadetector running on Google Colab GPU nodes can process it. Unfortunately, with typical upload speeds, it takes a long time to do the easy thing, which is to transfer 10’s of GBytes/SD card up where it can be processed. A useful system could be built by taking a few shortcuts, namely: reducing the resolution of each image to the native resolution of MegaDetector; and processing every Nth frame from the video (instead of all frames). This would all have to be done locally, but it would substantially reduce the amount of data that had to be shipped up into the cloud
I’ve prototyped this whole sequence, and it all works, in principle. The prototype is very slow (does not include all the shortcuts, above. But it’s a straightforward integration of existing tools. But I’ve been working on other things, and haven’t gotten around to packaging it up.
-bob
While trying to capture images of a raccoon on a garage roof using a trail camera, the only images I was able to capture occurred in the early morning and they were ghost-like. This I attribute to the high background temperature of the roof. I might have had much better results on a cold day.
What do you mean by “ghost like” ? (sorry that WordPress doesn’t allow photo attachments in comments 🙁 ) Black and white? Underexposed? Overexposed? Mirage like? Is the roof really that hot in early morning? Let me know if you’d like me to look at the photo, and I’ll contact you by email for a copy. Or let me know if it’s available somewhere online (e.g. FB)